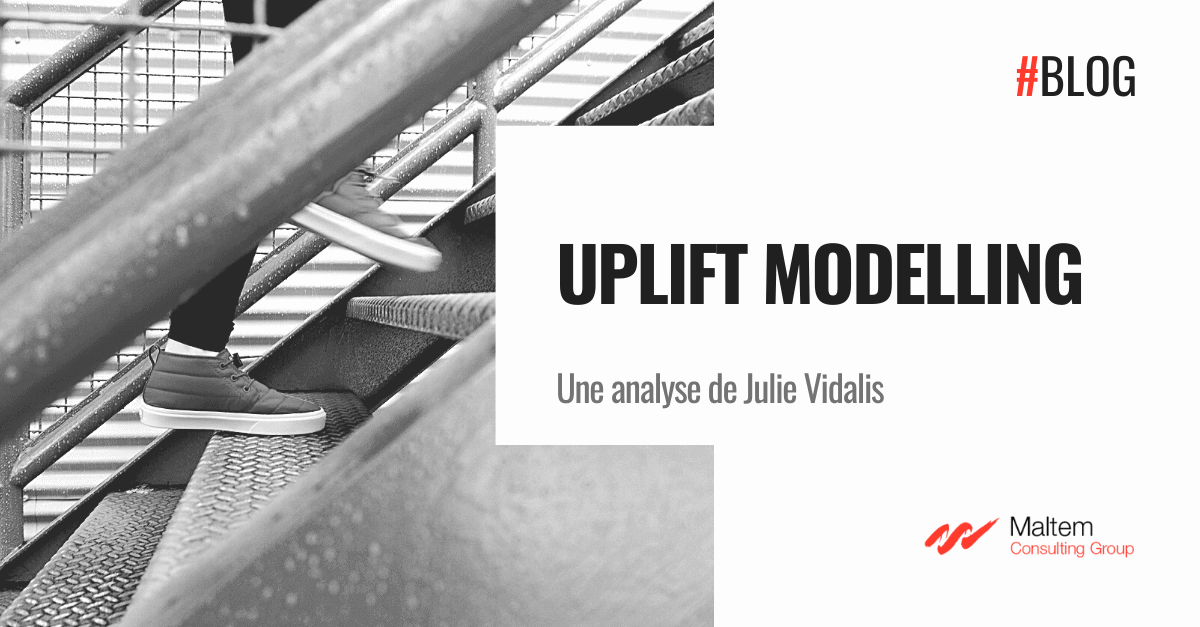
Uplift modelling, une analyse de Julie Vidalis
Lorsque l’on considère les mesures de performance des entreprises, les indicateurs financiers, c’est-à-dire la croissance du chiffre d’affaires et la rentabilité, sont les plus utilisés. Et les conseils d’administration et les actionnaires continuent à les utiliser dans le cadre du triple bilan. Ces indicateurs sont étroitement liés à l’amélioration des mesures de la satisfaction des clients, à l’accroissement de l’innovation, à l’amélioration des coûts directs et indirects, à la diversification des sources de revenus et à d’autres mesures bien documentées et étudiées qui ont un impact direct sur la croissance et la durabilité des entreprises.
Le cabinet de conseil IT vous présente :
QUANTIFIER L’AUGMENTATION DES VENTES AU DÉTAIL LORS DE LA MODIFICATION DE LA GAMME DE PRODUITS
Par Julie Vidalis
Lorsqu’une entreprise poursuit sa trajectoire de croissance, la complexité de son assortiment de produits est souvent introduite alors qu’elle ajuste son modèle de revenus et de coûts. C’est particulièrement le cas dans le secteur de la vente au détail, où une entreprise peut avoir plusieurs dizaines de milliers de marques différentes et de variations de gammes de produits proposées.
Considérons le secteur ci-dessus, avec un exemple d’une entreprise de vente au détail dont l’objectif est d’augmenter le chiffre d’affaires total et la rentabilité par magasin, en ajustant son assortiment de produits dans ces magasins individuels. Un mauvais assortiment de produits a une incidence sur l’expérience d’achat du client et peut entraîner une perte de ventes, tant directes qu’indirectes. Cette responsabilité incombe souvent aux Category Managers, qui doivent comprendre l’impact réel des décisions de révision de leur gamme de produits sur la satisfaction des clients et, en fin de compte, sur les ventes et la rentabilité du magasin.
Les raisons d’une augmentation des ventes ne peuvent pas toujours être mesurées directement, car de multiples facteurs peuvent influer sur une augmentation ou une diminution des ventes. Les ventes observées après l’introduction d’une nouvelle gamme de produits en magasin ne sont pas nécessairement le résultat exclusif d’une modification de la gamme de produits. La COVID-19 est un cas d’espèce, où un facteur externe important, qui échappe au contrôle des détaillants, a affecté des milliers de ventes en magasin, indépendamment des décisions prises en matière d’assortiment de produits.
Vous cherchez un cabinet de conseil IT pour utiliser vos données comme levier de performance ? Maltem est la solution. Contactez-nous pour obtenir un devis gratuit.
La solution est d’utiliser la modélisation par élévation.
L’objectif de la modélisation ascendante est de prédire l’impact différentiel d’un changement unique, ou d’un traitement, sur une variable dépendante spécifique. En utilisant la modélisation prédictive, en isolant l’effet d’un changement dans une variable indépendante, cette approche est basée sur le modèle d’inférence causale de Rubin qui stipule que :

Où Y est la variable de résultat (dépendante).
Une approche courante pour quantifier l’effet causal est l’utilisation de l’approche traditionnelle. Cette approche utilise les ventes observées après la mise en œuvre de la combinaison de produits comme ?(traité). Un algorithme de prévision par apprentissage automatique est utilisé pour prédire les ventes afin de simuler ce qui aurait pu se passer si la gamme de produits était restée la même et devient ?(traité). L’augmentation finale est calculée en soustrayant les ventes observées des ventes prédites.

Toutefois, cela ne représente pas nécessairement une représentation exacte de l’augmentation des ventes. Le principal inconvénient de cette approche est qu’il n’existe aucun moyen concret de montrer que la modification de l’offre de produits était la seule variable affectant les ventes.
L’approche de modélisation de l’élévation a été développée pour remédier à l’inconvénient de l’approche traditionnelle qui ne prend aucune mesure pour isoler l’effet d’un seul changement. De nombreuses recherches ont été menées sur la modélisation ascendante utilisée dans les problèmes de classification, mais elle n’est pas aussi couramment utilisée dans les analyses de régression comme l’évaluation des ventes.
Une méthode qui constitue une adaptation positive à la modélisation ascendante pour un problème d’analyse de régression est l’approche du modèle unique avec indicateur de variable de traitement.
Dans le cas d’un détaillant qui modifie sa gamme de produits, le changement qui est introduit est un changement de gamme de produits et le paramètre de mesure est le chiffre d’affaires, qui est une variable dépendante continue. Les données de ventes utilisées sont regroupées selon qu’elles ont été collectées avant ou après la mise en œuvre du changement de gamme de produits, et sont identifiées dans l’ensemble de données par une variable indépendante appelée indicateur de traitement.
Dans cette approche, un algorithme de prévision par apprentissage machine est formé sur un sous-ensemble de données, où les données sont obtenues à la fois avant et après la mise en œuvre de la nouvelle gamme de produits pour une catégorie. L’un des indicateurs sur lesquels le modèle est formé est une variable de traitement. Cette variable agit comme un drapeau pour mettre en évidence les points de données qui proviennent de la pré et de la post-mise en œuvre. Pour cet exemple, supposons que la variable de traitement est appelée « indicateur post-mise en œuvre ». Elle est fixée à un pour tous les points de données recueillis après la mise en œuvre de la nouvelle gamme de produits, et à zéro pour les points de données recueillis avant la mise en œuvre de la nouvelle gamme de produits.
Un seul modèle formé est utilisé pour prédire les ventes deux fois. Le premier ensemble de prévisions est généré pour les ventes, tout en maintenant les drapeaux de post-mise en œuvre à un ou zéro. Ce processus est répété, mais toutes les variables de traitement sont d’abord mises à zéro pour représenter l’avenir alternatif si le changement de gamme de produits n’a jamais eu lieu.

Ce faisant, le modèle ne prend en compte que l’effet de la modification d’une seule variable indépendante. Dans ce cas, il considère l’effet de l’introduction d’une nouvelle gamme de produits sur les ventes. La somme de la différence entre la première et la deuxième série de prévisions devient le montant des ventes en hausse.
Même si divers facteurs doivent être pris en compte lors de l’élaboration du modèle pour s’assurer que le signal ascendant n’est pas perdu, c’est une excellente méthode pour isoler l’effet d’une décision qui a été mise en œuvre et donc mieux comprendre comment ces décisions ont eu un impact sur l’entreprise.